Financial Services Analytics (FSAN) (Ph.D.)
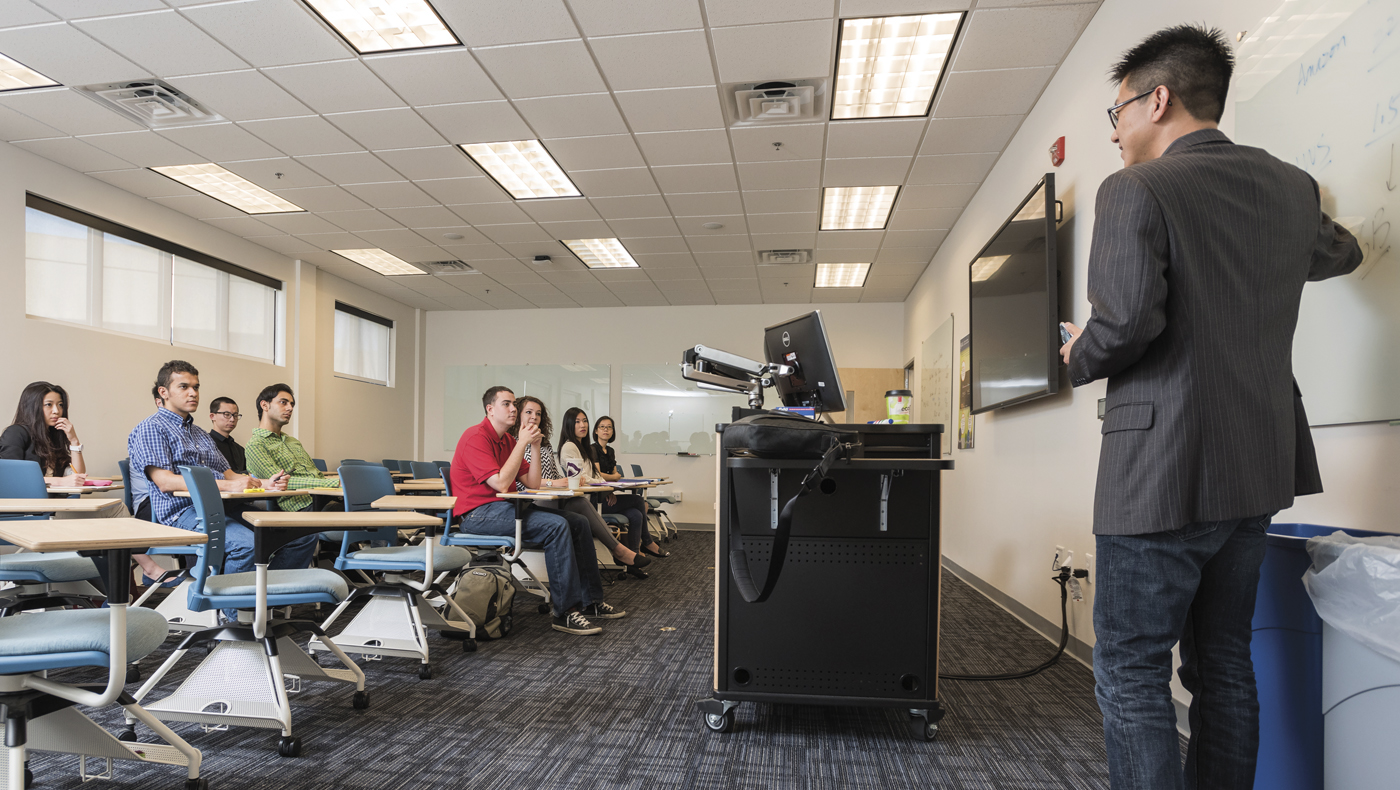
The financial services analytics (FSAN) doctoral program at the University of Delaware (UD) is the first of its kind, developing fundamental data science theories and models, and creating data-driven decision-making tools applicable to the financial services industry and other technology industries. It is an interdisciplinary STEM-designated program – a joint effort between UD’s Alfred Lerner College of Business and Economics and College of Engineering.
FSAN students have very diverse academic backgrounds; they have B.S. and/or M.S. degrees in areas such as math, statistics, computer science and many related business and engineering disciplines.
Program Highlights
Facilities
Our state-of-the-art facilities are designed to foster collaboration among students, faculty and industry; these include the JPMorgan Chase Innovation Center, the Geltzeiler Trading Center and the Harker Interdisciplinary Science and Engineering Laboratory.
Financial Support
Through a significant grant from JPMorgan Chase and Co, top candidates are provided with financial support – including tuition and a competitive stipend.
Student Experience
The small student cohort provides ample opportunities for close interaction with thought-leading faculty from multiple disciplines including business, engineering and computer science.
Program Requirements
To be granted a doctorate in FSAN, you must successfully complete all FSAN coursework with a 3.0 cumulative GPA. Most students complete all degree requirements within 4 to 6 years.
Courses (45-54 credits)
BUAD 640 – Ethical Issues in Domestic and Global Business Environments
FSAN 815/ELEG 815 – Analytics I: Statistical Learning*
FSAN 820 – Foundation of Optimization
FSAN 830 – Business Process Management Innovation
CISC 683 – Introduction to Data Mining
FINC 841/FSAN 841 – Financial Services Markets*
FINC 842/FSAN 842 – Financial Services Risk Analytics*
FSAN 850 – Financial Services Analytics Seminar (6 credits)
FSAN 860 – Current Research Topics (0-9 credits)
FSAN 969 – Doctoral Dissertation (9 credits)
Examinations and Dissertation
A qualifying exam and research paper are required at the end of the first year. A dissertation proposal and oral defense must be completed by the start of the third year. The dissertation requirement includes the approval of both your written dissertation and oral defense. Full exam and dissertation details can be found on myLerner.
A Look Into the Program
Student Research Topics
FSAN students work closely with their supervisors to identify cutting-edge research topics related to modern data science theory, algorithms and applications in banking and the financial and tech industries. Sample dissertation topics include:
- Bayesian causal inference
- predictive analytics
- bank systemic risk
- malware detection and classification
- credit card fraud detection
- NLP & chat-bots
- link recommendation
- text-based industry classification
- loan process optimization
- customer targeting
- active learning on graphs
Career Opportunities
Our program generates excellent internship opportunities and industry and academic job prospects.
Corporate-sponsored internships offer you the opportunity to apply analytics tools to real-world challenges on teams in wealth management, machine learning, data architecture, investment banking, global finance and business management. Most students gain internship experience while pursuing their Ph.D. degrees.
FSAN graduates are researchers and professionals who play key roles in teams that bridge the financial services industry and data and operational sciences. They are now employed as data scientists in major banks, fintech companies and technology companies. The FSAN program works closely with Lerner Career Services to provide personalized, high quality career assistance.
Where Our Students Intern
- JP Morgan Chase & Co
- Barclays
- Sallie Mae
- Amazon
- HP
Where Our Graduates Work
Academic Institutions:
- Chinese University of Hong Kong (Shenzhen)
- Shanghai University of Finance & Economics
- University of Delaware
- Virginia Tech
Banking and Financial Services:
- JP Morgan Chase & Co
- Bank of America
- Barclays
- Sallie Mae
- USAA
Industry:
- Amazon
- McKesson
- Meta
- Microsoft
"UD was able to merge finance, data mining, statistics and other areas to create the FSAN program. This is also reflected in the diversity of the students. We come from different fields, and it is wonderful how we are able to complement each other in so many different ways."
- FSAN student Leonardo De La Rosa Angarita
News
- Unique Lerner FSAN Program Lands Graduates in Top Financial Technology Jobs - As businesses increasingly search for workers skilled in tools like machine learning, artificial intelligence and language models, this program is turning out graduates ready for the job.
- What Is Fintech? - This article explains what fintech is, introduces jobs and salaries in the field and compares different ways of learning the subject.
- On the Job with Oliver Yao: New Lerner Dean Is Focused on the Promise of FinTech - Oliver Yao, the new dean of the Alfred Lerner College of Business and Economics, wants the college to be at the center of the financial technology revolution.
- Lifelong Lerner: Jared Sharpe - Jared Sharpe, a native of Andreas, Pennsylvania, earned a doctoral degree in financial services analytics from the University of Delaware’s Alfred Lerner College of Business and Economics in May 2023.
- Lifelong Lerner: Hongzhe Zhang - Hongzhe Zhang, a native of Jinan, China, earned a doctoral degree Ph.D in financial services analytics from the University of Delaware’s Alfred Lerner College of Business and Economics in May 2023.
Learn More
Contact Dr. Bintong Chen
302-831-2756
about-FSAN@udel.edu
Explore the doctorate in FSAN by viewing a recent FSAN information session.
Admissions Information
We accept students every other year, in the even-numbered years. The application deadline for the next cohort (beginning fall semester 2024) is May 1, 2024. Students seeking financial aid should submit their applications by February 1, 2024, but late applications will be considered if funds are available. Highly-qualified students may receive research scholarships as well as a generous stipend to cover all educational expenses.
Learn more about the financial services analytics Ph.D. admissions requirements, deadlines, tuition and financial aid available to you.
Institute for Financial Services Analytics (IFSA)
IFSA offers research seminars and conferences that connect education and industry in the emerging field of financial services analytics.
Carol A. Ammon Case Competition
Graduate student teams present their solutions to current issues faced by the competition’s focal company.